Is the time ripe for AI drug discovery?
Deep dive, Christoph Ruedig and Gita Kler
The current pressures on healthcare expenditure are unprecedented. The Covid-19 pandemic has led to a significant backlog of outpatient care. Within the UK, for instance, six million people are currently on NHS waiting lists – one in every nine people. Tackling this backlog will require additional resources. Longer-term trends such as demographic changes will continue to impact healthcare costs long after the pandemic has passed.
At the same time, the cost of medical advances has shot up. The UK’s spending on drugs has grown rapidly from £17.4 billion in 2016-17 to £20.9 billion in 2019-20. One reason for this is that innovative medicines are expensive to develop. The cost to bring new drugs to market has been doubling every decade to more than $2bn lately. Coupled with drug pricing pressures, patent cliffs and decreasing R&D productivity, pharma is experiencing a perfect storm.
AI and modern computing are helping other industries realise significant efficiency gains but have so far had a limited impact on pharma R&D productivity. That’s despite record amounts of funding in the AI drug discovery sector, which has grown from $1bn globally in 2015 to over $10bn in 2020. As one of the most active investors in European digital health and biotech, we believe that AI and modern computing, whilst not a panacea, holds the key to solving some of pharma’s biggest problems.
Pharmaceutical R&D Process
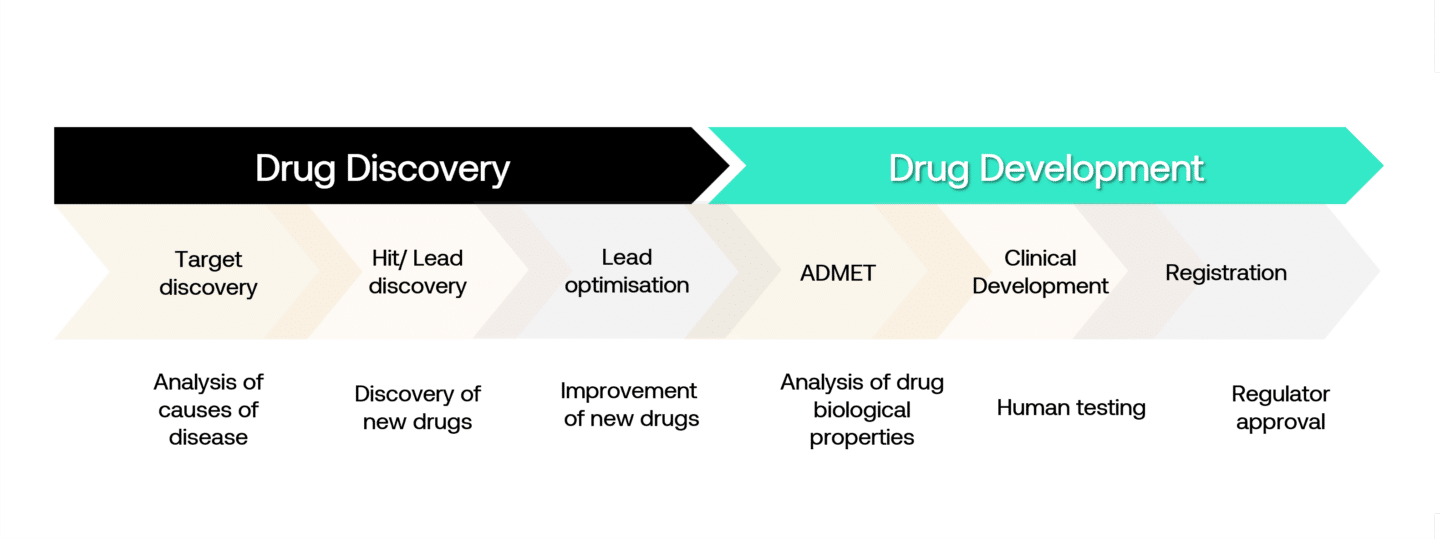
The pharmaceutical and biotechnology industry is one of the most research-intensive industries. An enormous amount of effort goes into the discovery and development of new medicines. In the ‘discovery’ portion, researchers spend time laboriously analysing the causes of disease to discover potential drug targets. These are often proteins representing central components in a pathway that isn’t working properly and which could be targeted via a drug. Once a target is identified, scientists try to find molecules that are highly specific to the target. Today, most of this is done “in vitro”, meaning in wet labs.
In the discovery of small molecules (typically chemical compounds), these in vitro experiments are done in high-throughput processes screening libraries of tens of thousands of compounds. For more complex biomolecules (peptides, antibodies, RNA) or advanced therapeutics (cell and gene therapies), the process is different partly because they are targeted and therefore don’t need to be optimised for specificity.
In vitro discovery is followed by “in vivo” studies, initially in animals where one or a small number of compounds with the best profile are tested for efficacy and toxicity. If a drug candidate is found to be safe and effective in animal trials, it is then progressed to clinical trials, initially in small populations of patients or volunteers to test for toxicity (phase I), followed by larger trials in patients to determine dosage and demonstrate efficacy and longer-term safety (phase II and III) before gaining approval.
The chart above shows the average time it takes to progress through drug discovery and development (X axis) and number of potential drug candidates (Y axis) for small molecule drug development. Despite the effort to choose the right drug candidate in the pre-clinical stages, failure rates in clinical trials are estimated to be roughly 90% on average, either due to safety issues or lack of efficacy. It is in the clinical stages, that costs compound rapidly. A Phase II clinical trial can add up to several million, while Phase III costs tens of millions. Dividing these costs by the chances of success and it becomes clear why drug development is so expensive.
The (potential) role of AI and modern computing in drug development
The idea of using computers to help in drug discovery and development isn’t new. The term computer-aided drug design (CADD) was coined in the 1980s. At the turn of the millennium, biotech VC firms began investing in a small number of “in silico” drug discovery companies. However, those initial efforts were largely unsuccessful. Compute power was simply too low, and AI was still in its infancy. As a result, the models used were not good representations of biology or chemistry.
Fast forward to today, and three foundational components have changed in favour of computational drug discovery. These include breakthroughs in the ability of computers to learn from large datasets in what is loosely termed artificial intelligence; the explosion in the amount of data, including genotypic, phenotypic and health record data; and the continued performance improvements in compute power and computing infrastructure. Researchers now have the tools to build models that better represent what is happening on a molecular, pathway and cellular level.
These technologies are extremely helpful in drug discovery. However, they will not eliminate the need for the most expensive parts of drug development: the in vivo testing in animals and human trials. How, then, can AI have a meaningful impact on R&D productivity? The answer is in both finding new and better targets and drugs.
New targets
One of the biggest challenges that researchers face is that many potential molecules in a diseased pathway are not “druggable”. This can be because they are hard to reach or because they have multiple functions leading to unwanted side effects if targeted (so-called “on-target side effects”). Computer models can support researchers by enabling faster druggability analyses that help determine whether a molecule warrants further resources or if it is time to pursue an alternative. They can help find workarounds to overcome complex druggability challenges by targeting other sites of the same molecule or finding other molecules in the same pathway that are more easily accessible or which, if targeted, show fewer side effects. In all these cases, researchers are trying to find the needle in a haystack. Computer models can help to narrow down millions of options to a few promising ones that can be explored in the wet lab, leading to better choices and shortening timelines.
New Drugs
Once a suitable target has been found, the biggest challenge is finding drug candidates that will be highly specific for the target but without affecting other parts of the body (“off-target side effects”). As explained earlier, during small molecule drug discovery researchers screen vast libraries of compounds to find a few that can be tested in vivo. Here, computational models can help find new chemical structures outside of the commonly used libraries, expanding the option space significantly in the hope of finding better drugs. They can also help with narrowing down the option space as explained above, again leading to better choices and shorter timelines.
Surfacing new targets and drugs enlarges the top of the funnel and the expectation is that this will ultimately lead to more drugs reaching the market. Equally, it is anticipated that models will have a meaningful impact on R&D productivity by enabling pharma companies to get better at choosing targets and drugs before they enter the clinical stages of the drug development cycle.
Besides these, there are other indirect ways by which modern computing can help R&D. For example, algorithms can help identify biomarkers by sifting through large volumes of publications and looking for associations between diseases and molecules that can be tested. These biomarkers can be useful for identifying the right patients to recruit into clinical trials, increasing the chances of success.
Proving that AI and modern computing can do all these things, however, will be challenging given the lead times and capital intensity of getting a drug through its many stages through to approval.
Technologies and commercial models used in AI Drug Discovery
How then are startups mobilising AI and modern computing? Startups are nimble, so we see a range of technologies and commercial models being pursued.
Technologies
Most (though not all) startups we have come across are using some form of machine learning on large datasets to train their models in understanding biology with the ultimate goal to make predictions about healthy and disease states and/or the impact of a perturbation “in vivo”. Datasets are either public or private and can span the gamut of DNA, RNA, proteins, cells, scientific publications, etc., all the way to medical records and patient-reported outcomes. Some companies have platforms that constantly generate new data, improving the models in a feedback loop. Some use high throughput “in vitro” experiments to create such data to ensure their models are always based on biology. Some companies go very deep into specific areas, for example, focusing only on certain molecules or therapeutic areas. Others are broader, scanning the universe of known drug compounds and disease states for drug repurposing.
Commercial models
A proportion of companies in the sector, such as Biorelate in the UK and Innoplexus in Germany, have adopted a pure software or fee-for-service model. These businesses are often trying to build large proprietary datasets and algorithms. There is a risk that, as data is commoditising, differentiation disappears. The other challenge is the size of the market for pure software or services today – Schrödinger, a well-established company in the USA, for example, has grown to about $100m of annual revenues in approximately 30 years, which highlights how small this market is.
Most companies, however, are adopting a partnership model, investing in proprietary compounds with ownership of the intellectual property (IP). These are then often licensed to pharmaceutical companies shortly before or after they have entered clinical trials. The advantage of this model is that it mostly avoids the expensive clinical development stages so resources can be focused on generating more early-stage programs. The trade-off is that ownership and control of the assets are given away. Also, lead times to generating significant revenues are long. However, the capital efficiency of this model is attractive, certainly in the early stages of a company, when there’s limited evidence of the value of the discovery engine.
Finally, a few companies, such as HealX in the UK and Pharnext in France, are embracing a more asset-centric model by focusing resources on developing their own drug pipelines into later clinical stages. These tend to look like classic biotech companies with broad capabilities across the R&D value chain.
European Market Map
We segmented European AI drug discovery startups in our market map according to their place in the drug discovery and development stage they primarily operate in plus their commercial models.
Concluding thoughts
AI drug discovery and development has been a long time coming. It is fantastic to see how much effort, money, technology, and talent have come into the sector over the last few years. We believe this is at the heart of the recent successes, as validated by startups striking lucrative partnerships with incumbent pharma companies.
Funding in the AI drug discovery sector has grown from $1bn globally in 2015 to over $10bn in 2020. The cost to bring new drugs to market has been doubling every decade to more than $2bn lately. Coupled with drug pricing pressures, patent cliffs and decreasing R&D productivity, pharma is experiencing a perfect storm.
Christoph Ruedig, Partner
To continue to be successful, startups need to focus on specific pain points along the pharmaceutical value chain. They also need to articulate their value proposition clearly to their clients and stakeholders. The pharmaceutical industry is very used to partnering. The biotech ecosystem has perfected value-creation in this industry through clever licensing and early-stage M&A. Learning how to “play this game” is perhaps the greatest challenge for the new crop of AI drug discovery entrepreneurs.
If you are a company or an investor active in AI drug discovery, or are generally interested we’d love to hear your thoughts hello@albion.vc.